The Role of Linkage Analysis in Delivering Positive Customer Experience for Casino Players
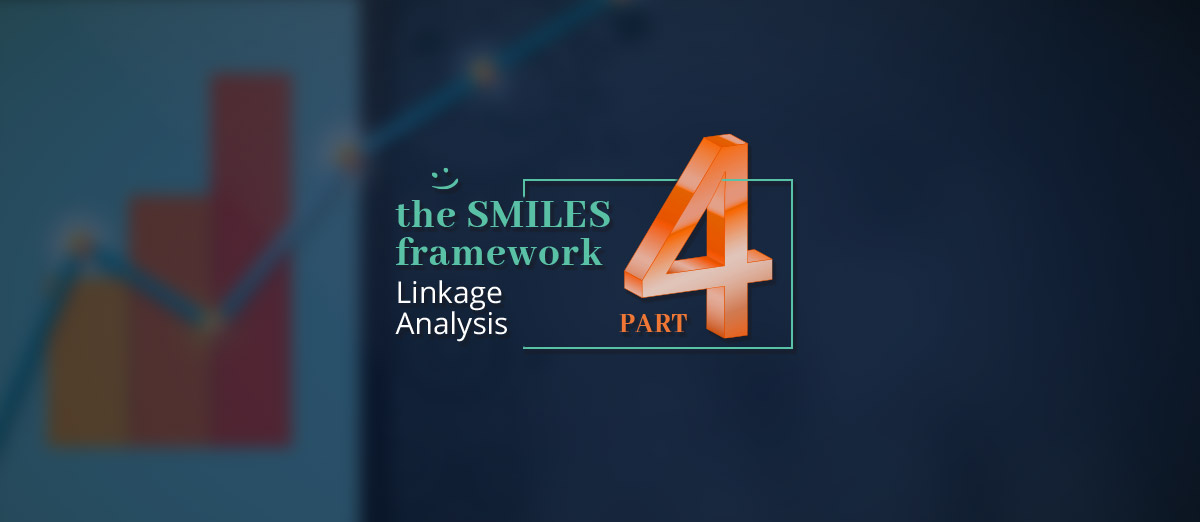
The effectiveness of customer experience (CX) efforts should ultimately be judged by the extent to which positive customer experiences lead to better business results. Most businesses struggle with proving the value of CX investments in terms of profitability and ROI. Consequently, it is difficult to get buy-in for CX projects from senior management. Linkage analysis enables businesses to quantify the value of customer experience in financial terms. Techniques used in linkage analysis also shed light on the interrelationships between operational, employee, and customer metrics, thus allowing businesses to understand the entire ecosystem of customer experience better.
Introduction
Customer experience is the outcome of a series of interacting variables, actions, and influences. Casinos are interested in CX because of its likely impact on growth, profitability, and market share. The task of CX leaders is twofold: (1) To understand the relationships between various causal variables and CX; and (2) To demonstrate the impact of CX quality on financial outcomes such as growth, profitability, and ROI. To accomplish these tasks, CX professionals use linkage analysis.
Linkage analysis, simply defined, is the process of combining and analyzing different sources of data to understand the relationships among their respective variables. It provides the intelligence needed to align people, processes, and activities such that the organization’s ability to make sound customer-based business decisions is enhanced.
The Linkage Analysis Process
The first step in linkage analysis is to determine exactly which questions you want to be answered through the analysis. A well-articulated research question (or set of questions) will guide decisions at each of the remaining stages in the process; for example, the time lag needed between the collection of predictor (independent) and outcome (dependent) variables data. An example of a clear research question would be, “Do table games customers report higher satisfaction in their interactions with casino dealers who have undergone customer-service training?” As shown in Figure 1, linkage analysis involves uncovering the relationships between data that often reside in disparate databases.
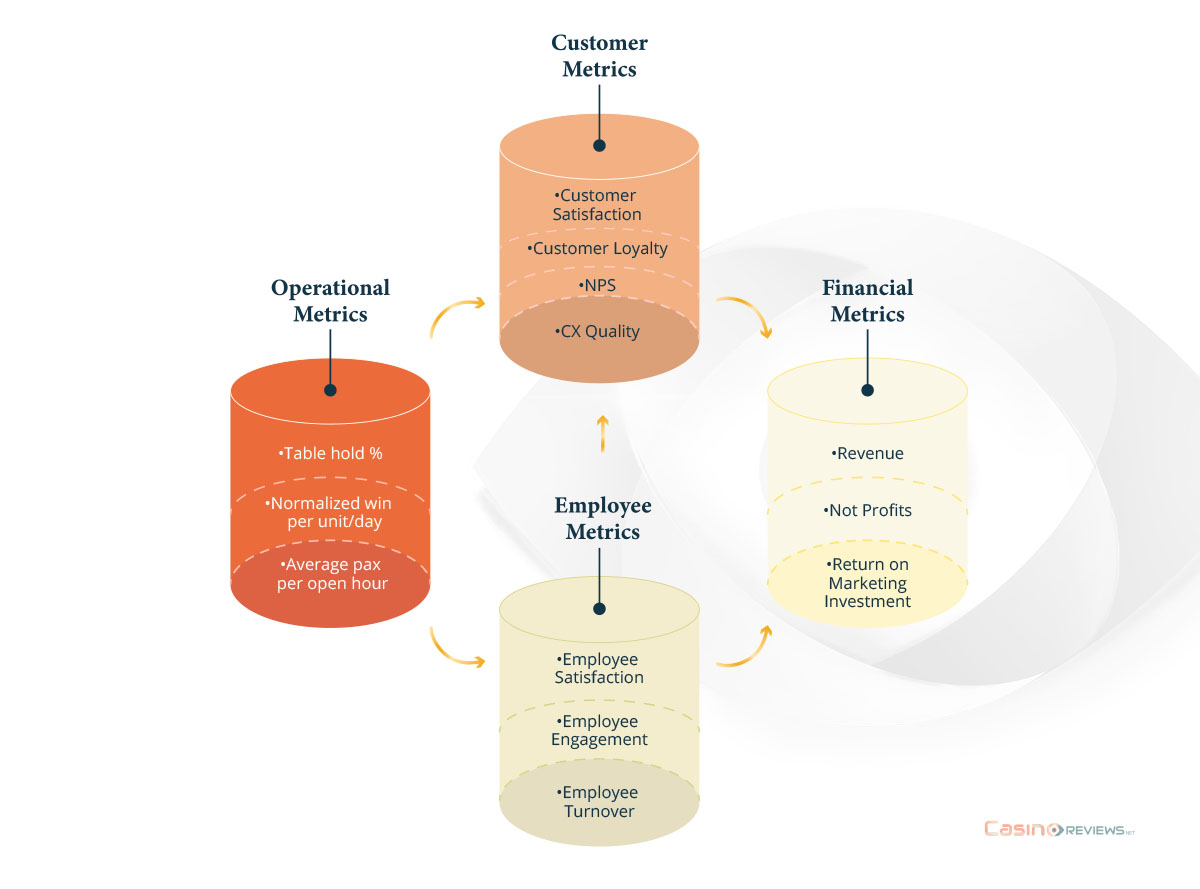
Figure 1: Disparate Databases Used to Generate Insights from Linkage Analysis
Upon deciding on one or more research questions to explore, the next step is to examine available data. One huge and often overlooked benefit of linkage analysis is that it highlights current gaps in a company’s data reservoir. Predictor and outcome variables used in linkage studies should ideally come from different databases.
However, it is not uncommon for less than adequately trained analysts to draw both the predictor and outcome variables from the same database, thus risking the analysis to common source bias and not allowing the time needed for the causal variables to have their impact felt on the outcome variables. Furthermore, in such a scenario, it is difficult to posit that one variable predicts another variable.
The units of analysis included in a linkage study (e.g., individual dealer or all dealers in a shift) are largely predicated on the availability of data and on the business owners of those data within organizations. For example, a casino may want to study the impact of background music on customer experience. This study might include data collected at both the individual customer level and shift level.
A decision now needs to be made whether to aggregate individual-level data to align with shift-level data (and risk losing valuable between-customer variability) or employ more sophisticated, multilevel analytical approaches. When deciding the unit of analysis, it is imperative that there exists significant variability for both the predictor and the outcome variables at the chosen unit of analysis.
The goal of linkage analysis is to figure out actionable leverage points to produce the desired outcome. Hence, if the units of analysis are not targeted at an actionable level, then linkage analysis becomes little more than an exercise in idle curiosity. For example, collecting customer data at the employee shift level would make sense if the shift manager had considerable leeway in deciding how the gaming floor is run during her shift. After deciding on the research questions to be answered, the data to be used, and the units on which the analysis needs to be based, the next step is to carry out data analysis.
The choice of technique used for data analysis depends on several factors such as the research questions to be answered, the number of cases in the dataset, and properties of the data (e.g., if the data to be analyzed are in nominal, interval, ratio, or ordinal format), and if the data are normally distributed. At its most basic level, simple means (averages) can be compared across two or more groups (e.g., time customers spend at the casino comparing customers who received a free meal offer versus those who did not).
It is not possible to discuss all the techniques that could be used in linkage analysis in this short article. However, any discussion on linkage analysis would be incomplete without a reference to machine learning. Machine learning techniques such as support vector machines are powerful modeling approaches for examining very large data sets with numerous variables. Recent customer experience platforms have built-in machine learning capabilities for linkage analysis. Figure 2 illustrates the model-building process using machine learning.
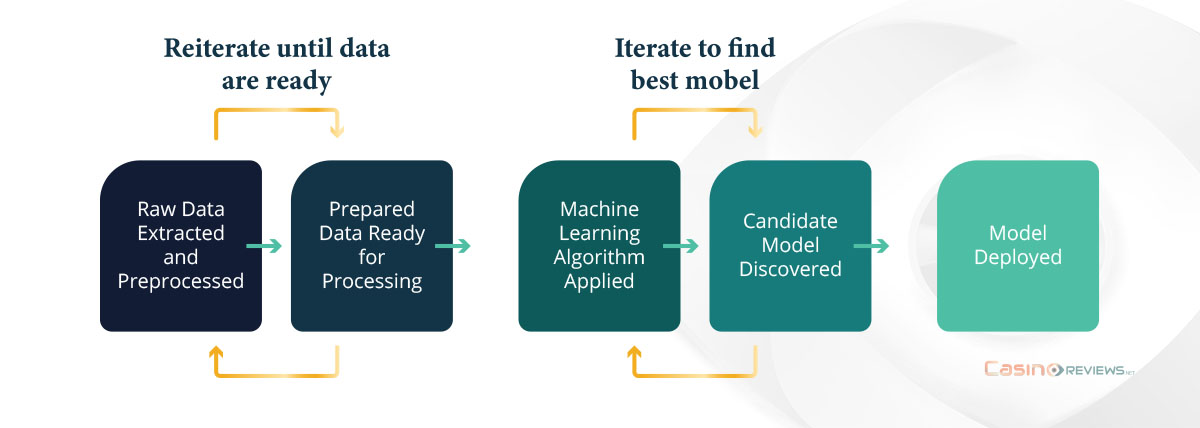
Figure 2: How Machine Learning Works
Applications of Linkage Analysis
Linkage analysis provides casino operators with the added intelligence needed to align people, processes, and activities, thereby enhancing the organization’s ability to make the right customer-based business decisions. In the realm of customer experience, linkage analysis is used to answer important questions related to the causes and consequences of CX efforts. Probably the most important application of linkage analysis is to provide a quantitative financial rationale for implementing CX initiatives.
A cost-benefit analysis of the CX program empowers the CX team to understand better underlying relationships between CX quality, customer retention, loyalty, and profitability. This vital intelligence sheds light on how CX ties to overall business wins. Linkage analysis is often used to calculate how customer experience relates to loyalty, how business operations are affecting retention, and the financial impact of CX improvements. These kinds of analyses help in making a solid case for CX investments.
One of the key barriers to CX programs is the lack of buy-in from senior management. The C-suite is often skeptical of the value of CX efforts. This is where linkage analysis comes to the rescue. By linking customer metrics to financial metrics, linkage analysis can convincingly demonstrate the ROI of various customer experience enhancing projects, thus connecting CX investments to the bottom line.
Conclusion
CX professionals are often asked to answer questions such as:
- What impact does changes in employee engagement have on customer satisfaction and loyalty?
- What impact do changes in customer satisfaction and loyalty have on the company’s financial performance?
- How does customer satisfaction impact the share of customer wallet?
- What impact does investment in internal marketing activities have on the customer experience?
- What impact would a one-point increase in NPS (Net Promoter Score) have on the company’s revenues?
Linkage analysis provides the intelligence needed to answer these and other vital questions. By analyzing different sources of data and uncovering the relationships between key operational, employee, customer, and financial metrics, linkage analysis takes most of the guesswork out of CX planning and execution. Advances in machine learning have made linkage analysis faster and more accurate. All that is needed are clean and relevant data and knowing the right research questions to ask.
Read more:
- How to Provide Your Customers the Best Casino Experience – The SMILES Framework
- The Role of Segmentation in Customer Experience
- Market Orientation and Value-Based Customer Experience in Casinos
- Internal Marketing – The Key to Delivering Great Customer Experience in Casinos
- The Role of Emotional Competence in Delivering and Improving Casino Customer Experience
- The Role of Service Blueprinting in Creating High-Quality Customer Experiences in Casinos
Review this Blog
Leave a Comment
User Comments
comments for The Role of Linkage Analysis in Delivering Positive Customer Experience for Casino Players